During the past, the realm of artificial intelligence (AI) was largely confined to the world of supercomputers and data centers. These powerful machines, with their immense computational capabilities, were the only entities capable of running sophisticated AI algorithms. However, the landscape of AI has evolved dramatically. Today, we find ourselves at the cusp of a remarkable transformation. Thanks to advancements in technology and the development of specialized hardware. We can now run powerful AI algorithms right on our smartphones. This remarkable achievement has ushered in the era of “Tiny AI”. Where the potential for AI-driven applications on our handheld devices seems boundless.
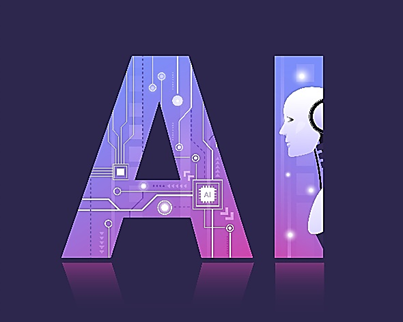
1. The Evolution of AI
To understand the significance of Tiny AI, it’s essential to appreciate the evolution of AI technology. AI, which was once relegated to the realm of science fiction, has rapidly advanced over the past few decades. From expert systems and rule-based AI to machine learning and deep learning. In terms of both capabilities and applications, AI has advanced significantly.
Initially, AI algorithms required massive computational power and access to extensive datasets to achieve meaningful results. This led to the development of AI that was largely centralized relying on data centers filled with powerful servers. These data centers became the beating heart of AI. Whereas, massive amounts of data were processed and analyzed, leading to breakthroughs in fields like natural language processing, computer vision, and more.
However, there was a fundamental limitation to this centralized AI paradigm. It could not operate in real-time or on personal devices. Running AI models on smartphones, for example, was impractical due to their limited computational resources and memory. As a result, the dream of having AI capabilities on our handheld devices remained elusive until recently.
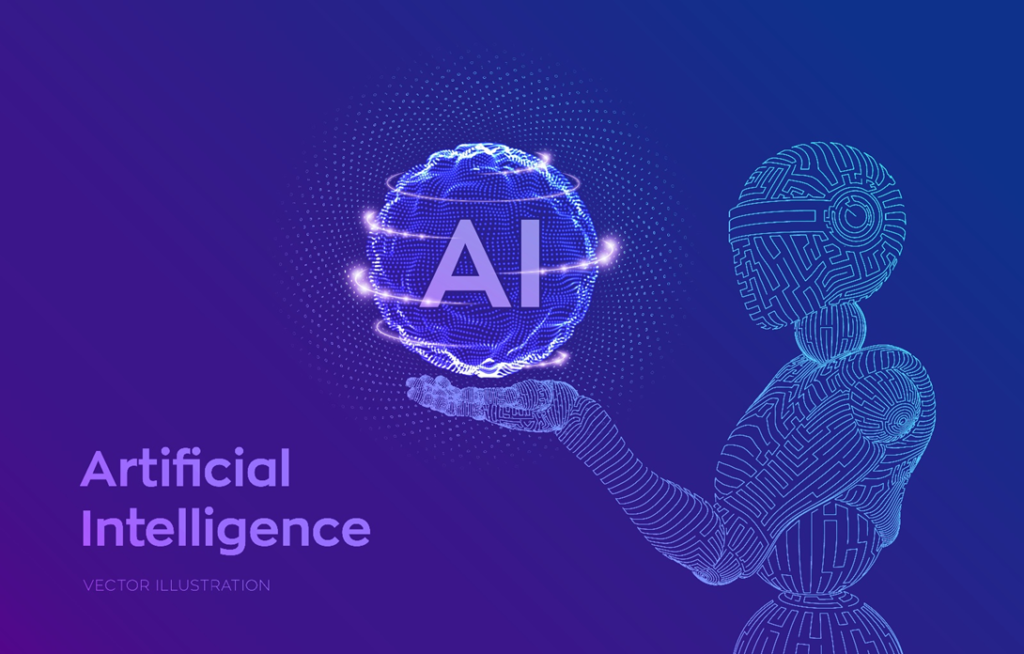
2. The Rise of Tiny AI
The breakthrough that paved the way for Tiny AI was the development of specialized hardware accelerators. Particularly, Graphics Processing Units (GPUs) and later, Tensor Processing Units (TPUs). These chips were designed to handle the complex calculations required by AI algorithms efficiently. This shift from general-purpose processors to specialized hardware marked a turning point in the AI landscape.
Tiny AI is all about taking advantage of these hardware accelerators to bring AI capabilities. It came in small, power-constrained devices like smartphones, tablets, and IoT devices. Here are some key elements that have contributed to the rise of Tiny AI:
2.1. Efficient Model Architectures
One of the key challenges in bringing AI to mobile devices was the size of AI models. Traditional deep learning models such as those used in natural language processing and image recognition. These were too large to run on smartphones. However, researchers have made significant progress in creating efficient model architectures. It delivers impressive performance while being compact enough to fit on mobile devices. For example, models like MobileNet and TinyBERT are designed specifically for resource-constrained environments.
2.2. Model Quantization
Model quantization is another critical technique in Tiny AI. It involves reducing the precision of numerical values used in neural networks. By using lower-precision data types (e.g., 8-bit integers instead of 32-bit floating-point numbers). It’s model, become more compact and can be executed with significantly fewer computational resources. This optimization technique is crucial for running AI on devices with limited processing power.
2.3. On-Device Training
Traditionally, AI models were trained in data centers and then deployed to devices. However, on-device training has emerged as a game-changer for Tiny AI. This approach allows AI models to be fine-tuned directly on the device using user-specific data. This capability has significant implications for personalized AI applications. Such as, predictive text input and voice recognition.
2.4. Edge Computing
The concept of edge computing involves processing data closer to the source (i.e., on the device or at the edge of the network). It has gained prominence in the era of Tiny AI. Edge devices including smartphones can process data locally. Therefore, reducing latency and the need for constant internet connectivity. This is especially important for applications like real-time image recognition, where immediate responses are crucial.
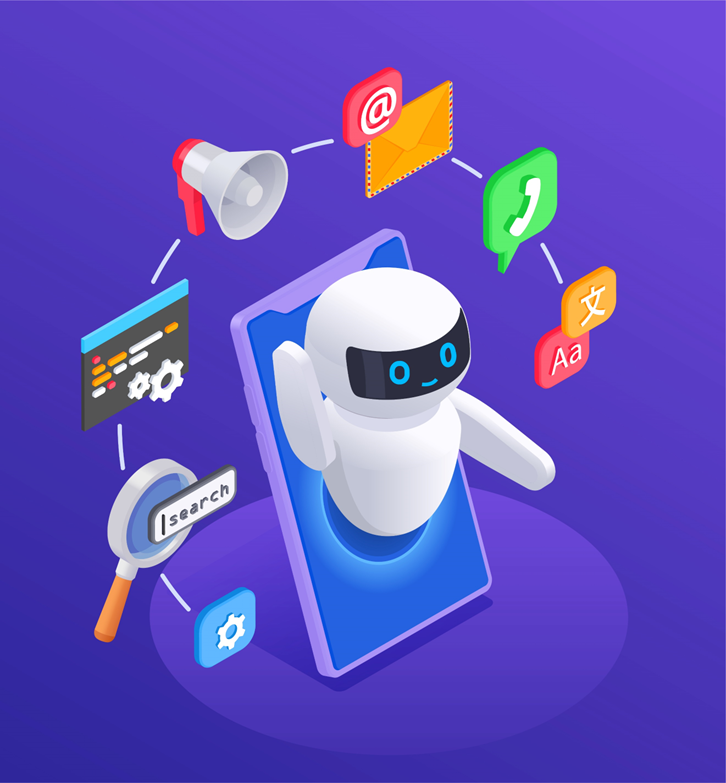
3. The Impact of Tiny AI
The advent of Tiny AI has had a profound impact on numerous industries and aspects of our daily lives. Let’s explore some of the key areas where Tiny AI is making a difference:
3.1. Personalized Experiences: Tiny AI enables personalized experiences on our smartphones. For instance, predictive text input and autocorrect algorithms use on-device AI to understand individual typing patterns and preferences. This results in more accurate and context-aware suggestions.
3.2. Voice Assistants: Voice assistants like Siri, Google Assistant, and Alexa rely on Tiny AI to understand and respond to spoken commands. The ability to process voice commands on the device itself which enhances privacy and responsiveness.
3.3. Healthcare: In healthcare, Tiny AI is used for applications like remote patient monitoring and early disease detection. Devices equipped with AI algorithms can analyze medical data. For example, real time ECG readings or X-rays allowing for rapid diagnoses and interventions.
3.4. Autonomous Vehicles: Tiny AI plays a crucial role in autonomous vehicles. On-board AI systems use real-time data from sensors to make split-second decisions. Keeping in mind the safety of passengers and pedestrians.
3.5. Augmented Reality: Augmented reality (AR) apps leverage Tiny AI to track objects and recognize the user’s environment. This enhances the immersive quality of AR experiences, whether for gaming or navigation.
3.6. Smartphones as AI Tools: Tiny AI has turned smartphones into versatile AI tools. Users can perform tasks like translating text, identifying objects and even measuring distances using AI-powered apps. All of these does not require an internet connection.
3.7. Privacy and Security: On-device AI processing enhances privacy by reducing the need to send sensitive data to cloud servers. Biometric authentication, such as facial recognition and fingerprint scanning, benefits from Tiny AI’s local processing capabilities.
4. Challenges and Future Directions
While Tiny AI has unlocked numerous possibilities. It also has its own set of difficulties and factors to take into account:
4.1. Resource Constraints: Despite optimization efforts, running AI models on smartphones can still strain computational resources and drain batteries. Balancing performance and power efficiency remains a constant challenge.
4.2. Data Privacy: As AI processing moves closer to the edge, ensuring data privacy becomes paramount. Protecting user data from unauthorized access and breaches is an ongoing concern.
4.3. Model Updates: Keeping AI models up to date on millions of devices can be a logistical challenge. Efficient mechanisms for model updates and version control are essential.
4.4. Standardization: The field of Tiny AI lacks standardized frameworks and tools. Establishing common standards can facilitate the development and deployment of AI applications across different devices.
4.5. Ethical Considerations: As AI becomes more integrated into our daily lives, ethical considerations regarding bias, fairness, and accountability become increasingly important. Developing ethical AI guidelines is essential.
4.6. Security: Ensuring the security of Tiny AI systems is crucial. These devices can be vulnerable to attacks and may become entry points for malicious actors if not properly protected. Developing robust security measures for Tiny AI is essential to prevent potential breaches and misuse.
4.7. Interoperability: Tiny AI applications and devices often come from various manufacturers and developers. Ensuring that these devices can seamlessly work together and share data in a standardized way can be a significant challenge. Interoperability standards need to be established to make these devices more user-friendly and effective.
4.8. Regulatory Compliance: As Tiny AI systems become more prevalent, regulatory bodies will need to catch up with the technology. Ensuring that these devices comply with existing regulations and that new regulations are developed. It is to address specific concerns related to Tiny AI is crucial for the technology’s responsible and widespread adoption.
4.9. Environmental Impact: The production and disposal of Tiny AI devices can have environmental implications. This includes the use of rare materials, energy consumption, and electronic waste management. Sustainable practices in the development and lifecycle of Tiny AI devices need to be addressed.
4.10. Education and Accessibility: While Tiny AI democratizes access to AI capabilities. There may still be barriers to adoption for certain communities. It may be else that the individuals with limited access to technology or technical knowledge. Efforts should be made to ensure that Tiny AI benefits. Which are accessible to a wide range of people and provides education and training available for its use.
4.11. Bias Mitigation: Just like larger AI systems, Tiny AI can also be prone to bias in data and algorithms. Efforts to detect and mitigate bias in these systems are essential to ensure fairness and avoid reinforcing existing biases.
4.12. Long-term Sustainability: As AI models and technologies evolve rapidly, ensuring the long-term sustainability and compatibility. Providing services to Tiny AI devices and applications can be challenging. Strategies for maintaining and updating these systems over extended periods need to be developed.
Looking ahead, the future of Tiny AI holds tremendous promise. As hardware continues to evolve, with the emergence of even more powerful mobile processors. The dedicated AI accelerators, the capabilities of AI on smartphones will only expand. Moreover, the synergy between 5G networks and Tiny AI will further enable real-time AI applications that were once unimaginable.
Tiny AI represents a significant milestone in the journey of artificial intelligence. It has democratized access to powerful AI capabilities bringing them into the palms of our hands. This transformation has far-reaching implications from personalized user experiences to advancements in healthcare, autonomous vehicles, and environmental conservation efforts.
With the proliferation of Tiny AI, we are witnessing a profound shift in how AI technologies are integrated into various aspects of our lives. These miniature yet mighty AI systems enable seamless automation, improved decision-making, and enhanced efficiency across industries and applications. As Tiny AI continues to evolve and become more accessible, it holds the potential to revolutionize everything. From agriculture and education to entertainment and beyond, ushering in a new era of innovation and opportunity.
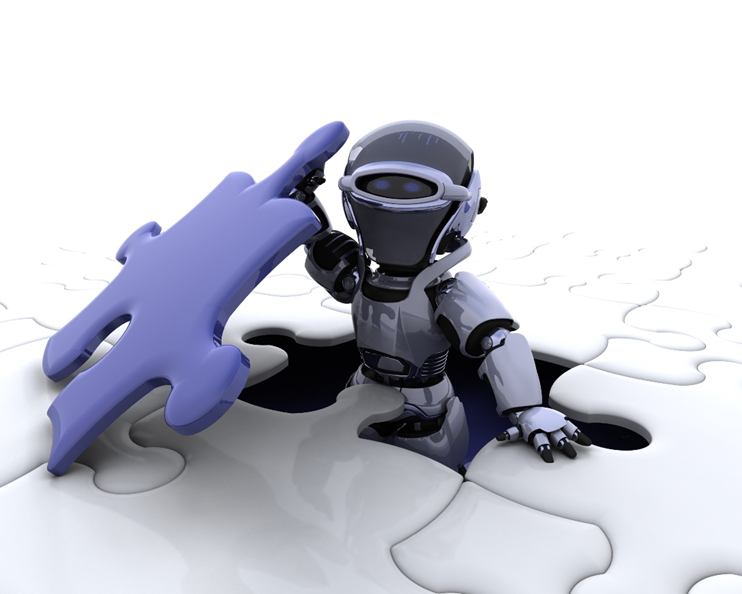